A gaze driven fast-forward method for first-person videos
Sixth International Workshop on Egocentric Perception, Interaction and Computing at the IEEE/CVF Conference on Computer Vision and Pattern Recognition (EPIC@CVPR) 2020
![]() |
AbstractThe growing data sharing and life-logging cultures are driving an unprecedented increase in the amount of unedited First-Person Videos. In this paper, we address the problem of accessing relevant information in First-Person Videos by creating an accelerated version of the input video and emphasizing the important moments to the recorder. Our method is based on an attention model driven by gaze and visual scene analysis that provides a semantic score of each frame of the input video. We performed several experimental evaluations on publicly available First-Person Videos datasets. The results show that our methodology can fast-forward videos emphasizing moments when the recorder visually interact with scene components while not including monotonous clips.
|
Methodology
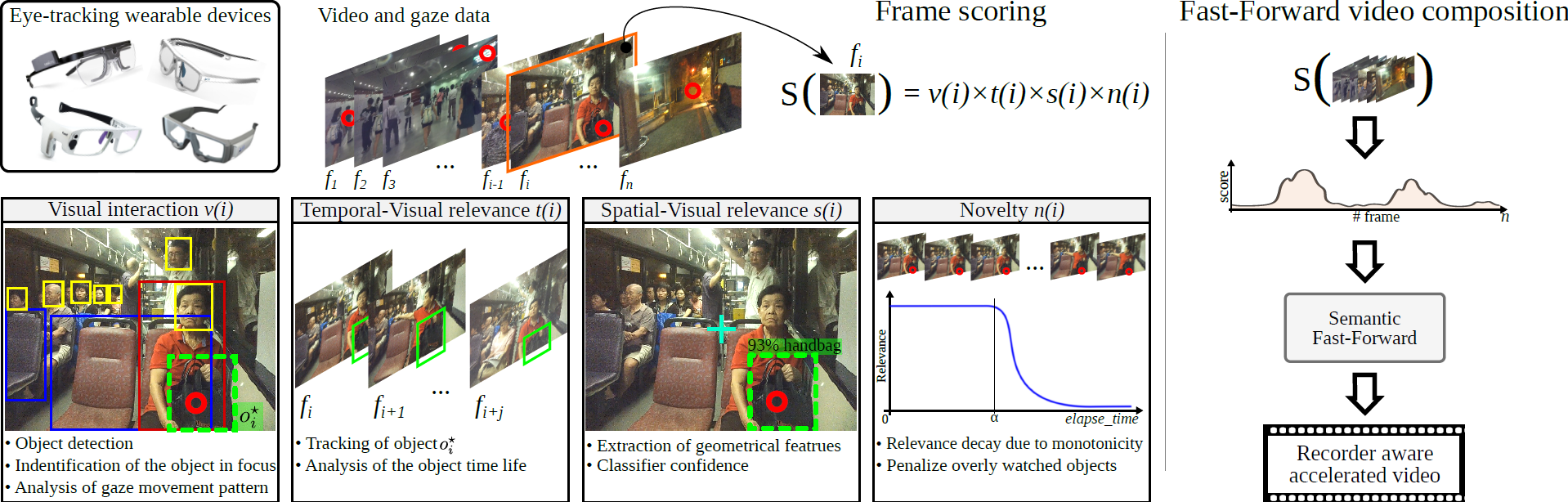
LinksSource code (Coming Soon!) ArXiv (NEW!) |
Supplementary Video with Visual Results |
Citation
@InProceedings{Neves2020epic@cvpr,
title = {A gaze driven fast-forward method for first-person videos},
booktitle = {Sixth International Workshop on Egocentric Perception, Interaction and Computing at the IEEE/CVF Conference on Computer Vision and Pattern Recognition (EPIC@CVPR)},
author = {Alan Neves, Michel Silva, Mario Campos, Erickson R. Nascimento},
Year = {2020},
month = {Jun.},
pages = {1-4}
}
title = {A gaze driven fast-forward method for first-person videos},
booktitle = {Sixth International Workshop on Egocentric Perception, Interaction and Computing at the IEEE/CVF Conference on Computer Vision and Pattern Recognition (EPIC@CVPR)},
author = {Alan Neves, Michel Silva, Mario Campos, Erickson R. Nascimento},
Year = {2020},
month = {Jun.},
pages = {1-4}
}
Baselines
We compare the proposed methodology against the following methods:
- Sparse Adaptive Sampling (SAS) – Silva et al., A Weighted Sparse Sampling and Smoothing Frame Transition Approach for Semantic Fast-Forward First-Person Videos, CVPR 2018.
- Multi-Importance Semantic Fast-Forward (MIFF) – Silva et al., Making a long story short: A Multi-Importance fast-forwarding egocentric videos with the emphasis on relevant objects, JVCI 2018.
Datasets
We conducted the experimental evaluation using the following datasets:
- ASTAR – available under request
- GTEA Gaze+ – publicity available at http://cbs.ic.gatech.edu/fpv/
Authors
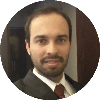
Alan Carvalho Neves
MSc Student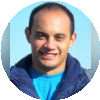
Michel Melo da Silva
Researcher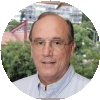
Mario F. M. Campos
Professor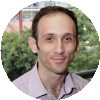