Semantic Description of Objects in Images Based on Prototype Theory
Abstract
This research aims to build a model for the semantic description of objects based on features detected in images. We introduce a novel semantic description approach inspired by the Prototype Theory. Inspired by the human approach used for representing categories, we propose a novel model that encodes, stores and retrieves the central semantic meaning of the object’s category: the prototype. The proposed prototype-based description model create discriminative descriptor signatures and describe an object highlighting its most distinctive features within the category. To achieve this goal, a mathematical model is developed to stand for and construct the semantic prototypes of object’s categories using Convolutional Neural Networks (CNNs). Our global semantic descriptor builds low-dimensional and semantically interpretable signatures that encode the semantic information of the objects using the constructed semantic prototypes. In our experiments, using publicly available datasets, we show that: i) the mathematical model of the proposed semantic prototype is able to describe the category internal structure; ii) the proposed semantic distance metric can be understood as the object typicality score within a category; iii) the global semantic descriptor representation preserves the semantic information used by the CNN classification models, and objects typicality score; iv) our semantic descriptor encoding significantly outperforms state-of-the-art global descriptors in terms of cluster metrics.
Methodology
Step 1 – Offline processing: Computing prototypes
Step 2 – Online processing: Global Semantic Description
Publications
[WACV 2019] Omar Vidal Pino, Erickson R. Nascimento, Mario F. M. Campos. Prototypicality effects in
global semantic description of objects, IEEE Winter Conference on Applications of Computer Vision (WACV), 2019.
Visit the page for more information.
This project is supported by CNPq, CAPES, and FAPEMIG.
Team
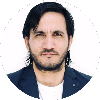
Omar Vidal Pino
PhD Candidate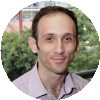
Erickson Rangel do Nascimento
Associate Professor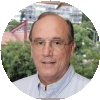