A Two-Step Learning Method For Detecting Landmarks On Faces From Different Domains
2018 IEEE International Conference on Image Processing (ICIP)
Abstract
The detection of fiducial points on faces has significantly been favored by the rapid progress in the field of machine learning, in particular in the convolution networks. However, the accuracy of most of the detectors strongly depends on an enormous amount of annotated data. In this work, we present a domain adaptation approach based on a two-step learning to detect fiducial points on human and animal faces. We evaluate our method on three different datasets composed of different animal faces (cats, dogs, and horses). The experiments show that our method performs better than state of the art and can use few annotated data to leverage the detection of landmarks reducing the demand for large volume of annotated data.
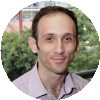
Coming Soon. |
Methodology and Visual Results |
Citation
@InProceedings{frade2018icip,
author = {Bruna V. Frade and Erickson R. Nascimento},
title = {A two-step Learning Method For Detecting Landmarks On Faces From Different Domains},
booktitle = {IEEE International Conference On Image Processing (ICIP)},
year = {2018}
}
author = {Bruna V. Frade and Erickson R. Nascimento},
title = {A two-step Learning Method For Detecting Landmarks On Faces From Different Domains},
booktitle = {IEEE International Conference On Image Processing (ICIP)},
year = {2018}
}
Datasets
[Kaggle] – Human Face.
Visit the original dataset page.
Download adapted dataset.
[CVPR 2011] – Dog Face.
Visit the original dataset page.
Download adapted dataset.
[Kaggle] – Cat Face.
Visit the original dataset page.
Download adapted dataset.
[CVPR 2017] – Horse Face.
Visit the original dataset page.
Download adapted dataset.
Authors
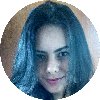
Bruna Vieira Frade
MSc Student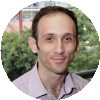