Leveraging Semantic Cues from Foundation Vision Models for Enhanced Local Feature Correspondence
Asian Conference on Computer Vision (ACCV 2024)
Visual correspondence is a crucial step in key computer vision tasks, including camera localization, image registration, and structure from motion. The most effective techniques for matching keypoints currently involve using learned sparse or dense matchers, which need pairs of images. These neural networks have a good general understanding of features from both images, but they often struggle to match points from different semantic areas. This paper presents a new method that uses semantic cues from foundation vision model features (like DINOv2) to enhance local feature matching by incorporating semantic reasoning into existing descriptors. Therefore, the learned descriptors do not require image pairs at inference time, allowing feature caching and fast matching using similarity search, unlike learned matchers. We present adapted versions of six existing descriptors, with an average increase in performance of 29% in camera localization, with comparable accuracy to existing matchers as LightGlue and LoFTR in two existing benchmarks.
Code and weights are available below!
LinksConference Paper (Coming soon!) |
Team
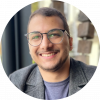
Felipe Cadar Chamone
PhD Student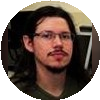
Guilherme Augusto Potje
PhD Candidate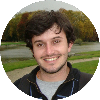
Renato José Martins
Professor at Université de Bourgogne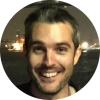
Cedric Demonceaux
Professor at Université de Bourgogne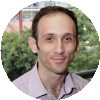